' À la carte ' - Workshops
Deep Learning | Tasks
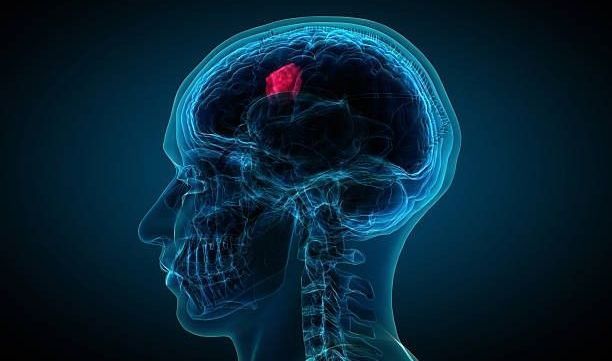
Prediction & Classification
Supervised machine learning algorithms are designed to enable predictions based on historical data, like training them to classify emails as spam or not spam, diagnose diseases from patient records, and even predict housing prices based on past real estate data.
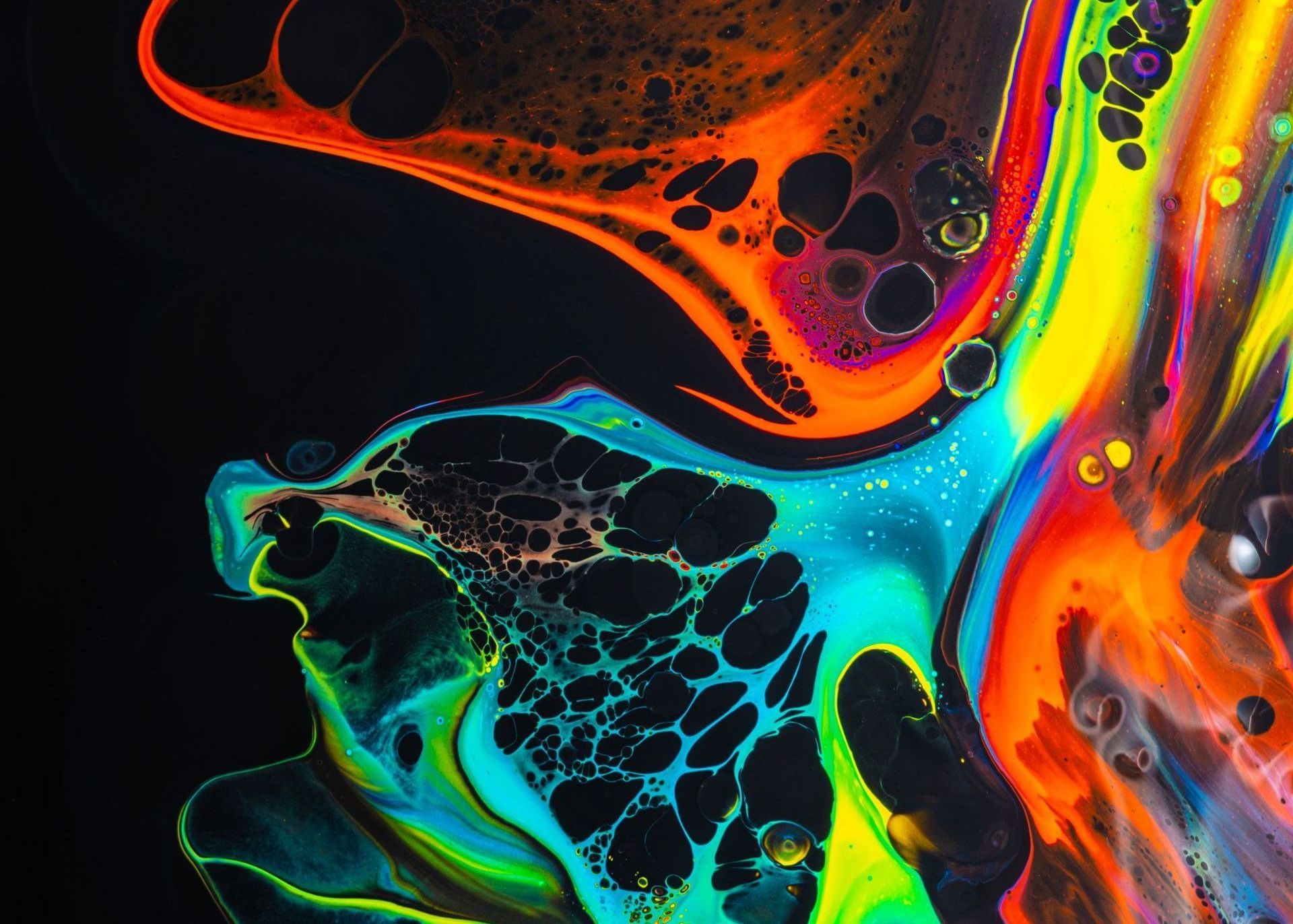
Generative AI, Clustering, and Dimensionality Reduction
Unsupervised machine learning techniques delve into the hidden patterns within data, allowing you to uncover insights without explicit historical labels – for instance, grouping similar customer purchasing behaviors for targeted marketing, generating new artificial 2D and 3D assets for virtual worlds, and reducing the dimensions of financial data for more efficient analysis.
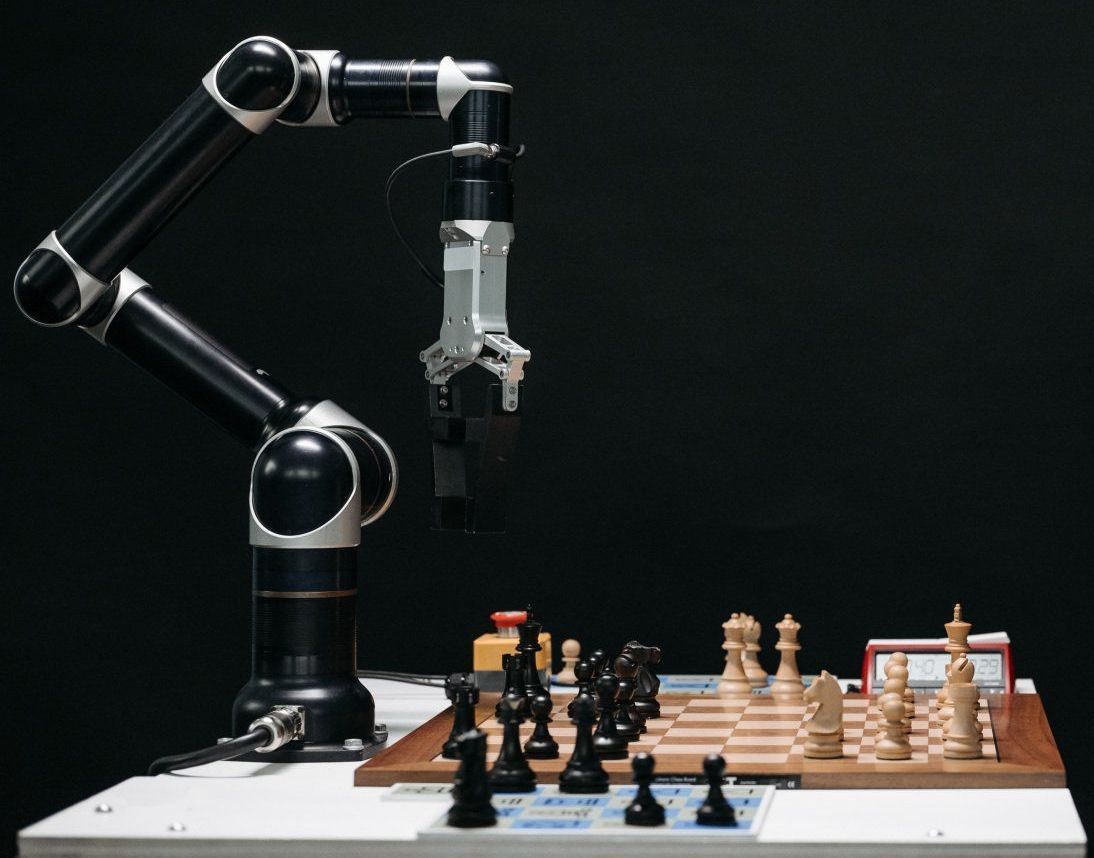
Optimal Sequential Decision Making
Reinforcement Learning, a dynamic branch of machine learning, focuses on training algorithms to make sequential decisions by interacting with an environment, much like teaching a robot to learn tasks such as optimizing a manufacturing process, mastering complex games like chess or Go, and even managing the energy consumption of a building to enhance efficiency.
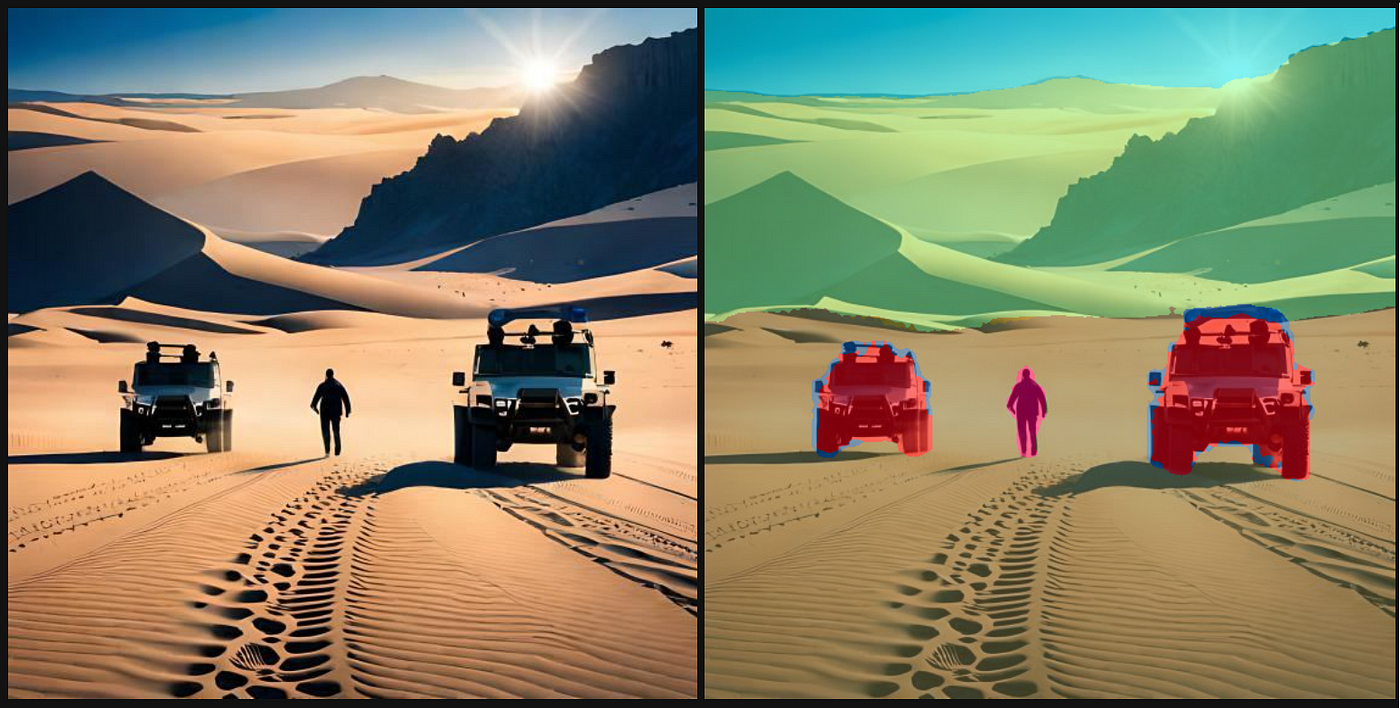
Automated Feature Extration
In the realm of Self-Supervised Learning, the emphasis lies in leveraging the inherent structure of data itself to create learning signal, enabling tasks such as training a model to predict missing words in sentences for language understanding, creating meaningful image representations without explicit annotations, and even pretraining models on vast amounts of unlabeled data before fine-tuning them for specific tasks, like object detection or sentiment analysis.
Deep Learning | Modalities
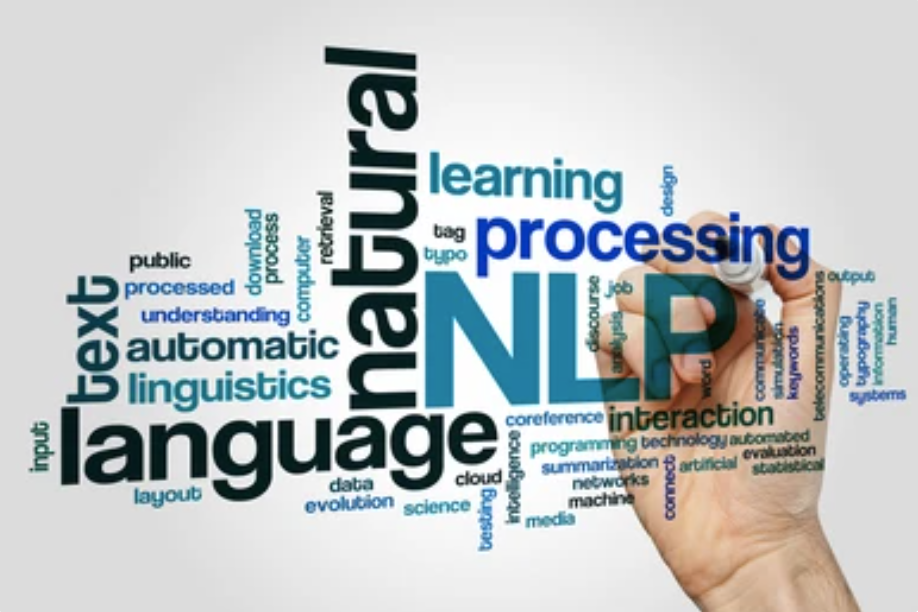
Analyze and Generate Natural Language
Within the field of Natural Language Processing (NLP), advanced algorithms enable computers to understand, interpret, and generate human language. This empowers machines to perform tasks like sentiment analysis, where they determine the emotional tone of a text; language translation, transforming text from one language to another; and even chatbot development, constructing conversational agents capable of engaging in meaningful interactions with users.
Leverage Image Data
Leveraging the expressivity of modern Deep Learning architectures, Computer Vision equips computers with the ability to interpret visual information from the world. It enables machines to identify objects in images through object detection, recognize individuals in photos using facial recognition, and even analyze medical images like X-rays and MRIs to assist in diagnosis. Through Computer Vision, technology gains the capacity to understand and process visual data, paving the way for applications ranging from self-driving cars to augmented reality experiences.
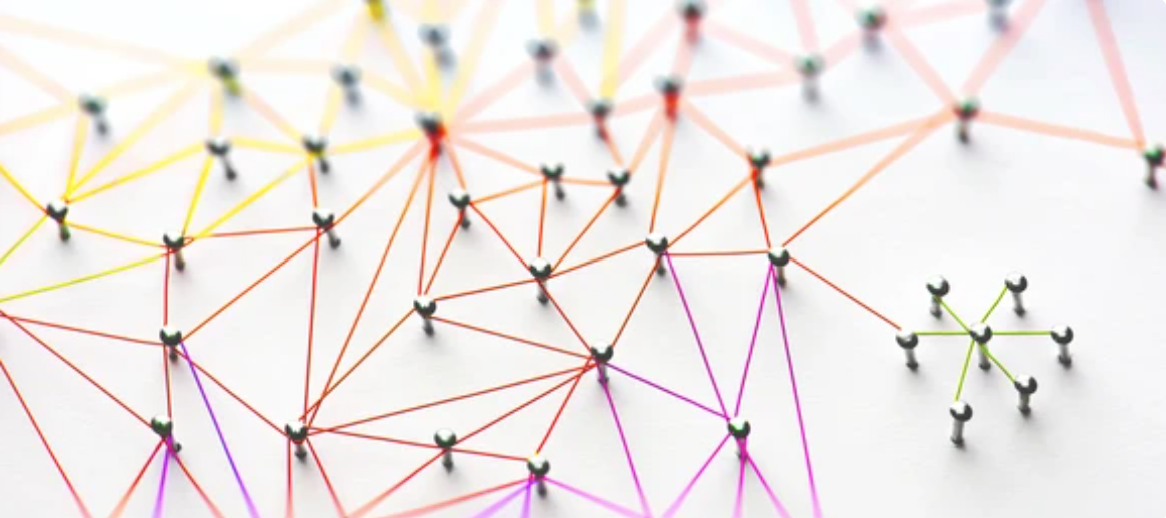
Fully Exploit Arbitrarily Complex Structures and Hierarchies
Graph Neural Networks (GNNs) form the bedrock of analyzing data structured as graphs. GNNs excel in scenarios like social network analysis, where they capture relationships between individuals, studying molecular structures to discover new drugs, and even recommending products based on interconnected user preferences in recommendation systems. By propagating information across nodes in a graph, GNNs unlock insights from intricate relational data that traditional methods struggle to uncover.
Deep Learning | Architectures & Inductive Biases
Exploit Local Spatial Patterns
By extracting hierarchical features from various types of structured data, convolutional neural networks excel in capturing local patterns, whether in images, videos, text sequences, or even molecular structures. They find applications in image analysis, natural language processing, and beyond, empowering machines to discern intricate relationships. These techniques remained the go to method for image exploitation (and still are in many cases) until Transformers emerged.
Exploit Sequential Patterns
Recurrent Neural Networks (RNNs) serve as a fundamental framework for processing sequential data across diverse domains. Whether in natural language processing for tasks like language generation and sentiment analysis, financial forecasting by analyzing time series data, or even speech recognition to transcribe spoken words into text, RNNs excel in capturing temporal dependencies and patterns. With their ability to retain memory of previous inputs, RNNs empower machines to navigate and understand sequences, making them a cornerstone in various applications requiring sequential understanding and context.
Attention Is All You Need
Transformers, a groundbreaking architecture in the realm of machine learning, revolutionize aggregation learning tasks by capturing global relationships and contextual information. They excel in natural language processing, enabling tasks like machine translation, text generation, and question answering, and are a key component of Large Language Models (LLMs) and modern conversational AI systems. Additionally, Transformers have found utility in computer vision for tasks such as image captioning and image classification, showcasing their adaptability across modalities. By leveraging self-attention mechanisms, Transformers process input data in parallel while maintaining a holistic understanding of context, making them indispensable in tasks that demand capturing intricate dependencies across vast datasets.
Other
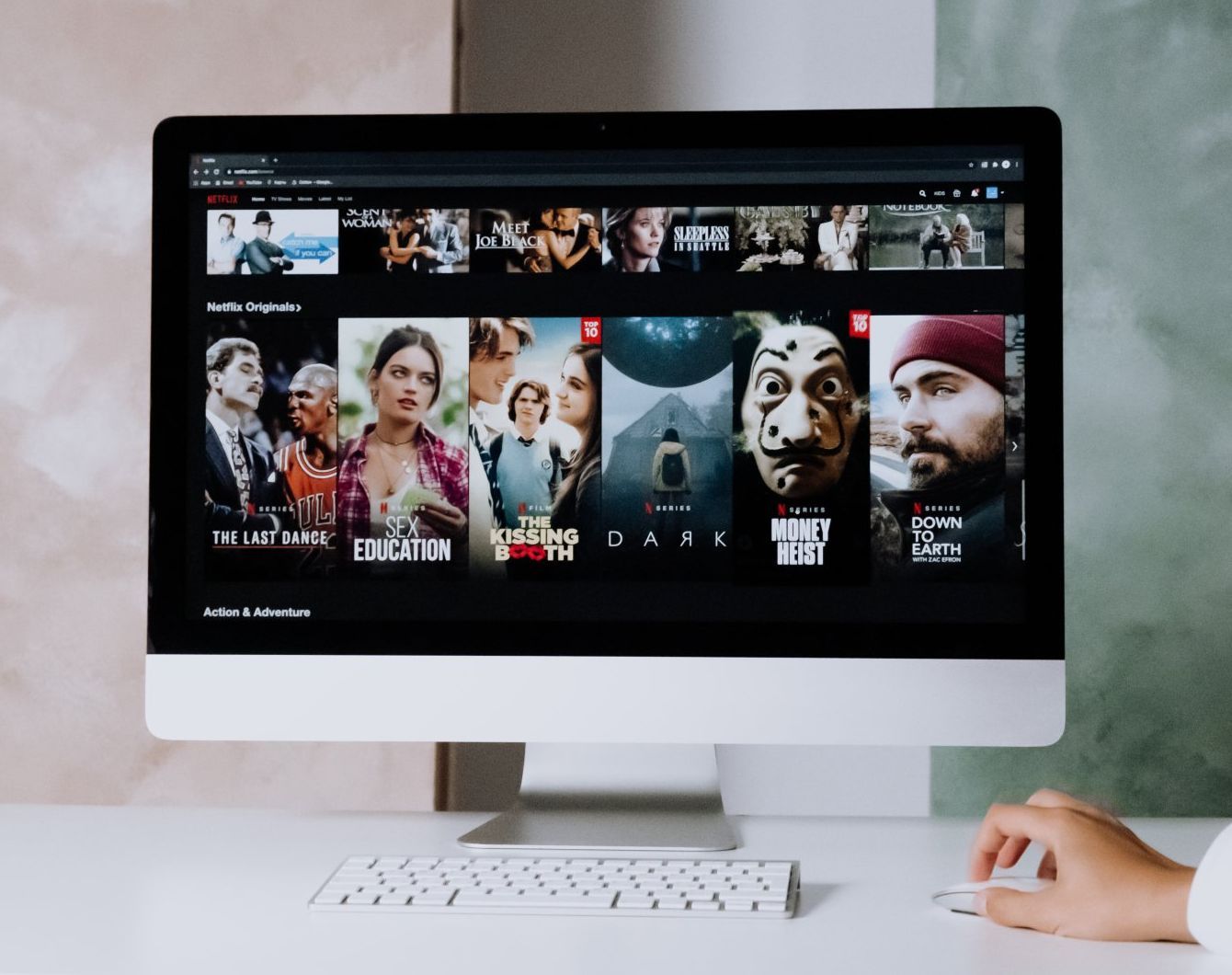
Finding Optimal User-Item Matches
Recommender Systems are sophisticated algorithms designed to predict and suggest items of interest to users. These systems play a vital role in diverse fields, such as e-commerce, streaming services, and content platforms. They leverage user behavior data to provide personalized recommendations, aiding users in discovering products, movies, music, or content that aligns with their preferences. By analyzing patterns of user interactions, such as purchase history or viewing habits, Recommender Systems enhance user engagement and satisfaction by offering tailored suggestions, thus optimizing the overall user experience.